EVALUACIÓN DE LA PRECISIÓN EN EL PRONÓSTICO DE LA INFLACIÓN EN BOLIVIA: RANDOM FOREST Y ÁRBOLES DE DECISIÓN VS. ARIMA
DOI:
https://doi.org/10.52428/20758960.v15i39.1227Keywords:
Inflation Forecasting, Machine Learning, Random Forest, Decision Tree, ARIMA Model, BoliviaAbstract
This study compares IPC forecasting models in Bolivia, evaluating traditional and machine learning approaches for inflation prediction. Decision Tree, Pruned Tree, Random Forest, and ARIMA models were applied, finding that machine learning models, particularly the Pruned Tree, outperform ARIMA in accuracy, achieving a lower RMSE in the test set. This suggests that modern models better capture the complex dynamics of the IPC and represent more robust tools for inflation projection in emerging economies. The study recommends exploring hybrid models and advanced neural networks in future research to further optimize forecasts.
Downloads
References
Banco Central Europeo. (2023). Quantile Regression Forests for Inflation Prediction in the Eurozone: Short-Term Adaptability in Forecasts. Banco Central Europeo.
Bojanic, A. N. (2021). A Markov-Switching Model of Inflation in Bolivia. Economies. DOI: https://doi.org/10.3390/economies9010037
Box, G. E., & Jenkins, G. M. (1970). Time Series Analysis: Forecasting and Control. Holden-Day.
Bratu, M., & Nicula, A. S. (2024). Sentiment analysis as an innovation in inflation forecasting in Romania. Marketing i menedžment innovacij, 15(2), 13-25. DOI: https://doi.org/10.21272/mmi.2024.2-02
Breiman, L. (2001). Random forests. Machine learning, 45, 5-32. DOI: https://doi.org/10.1023/A:1010933404324
Breiman, L., Friedman, J., Stone, C., & Olshen, R. (1984). Classification and Regression Trees. Chapman and Hall/CRC.
Carmona Rodríguez, C. S., & López Sánchez, A. (2021). Revisión de implementación de modelo de Random Forest en los últimos años. Universidad Pontificia Bolivariana.
Diaf, S., & Schütze, F. (2024). Economic forecasting with non-specific Google Trends sentiments Insights from US Data. 6th International Conference on Advanced Research Methods and Analytics (págs. 10-17). Valencia: Universidad Politécnica de Valencia. DOI: https://doi.org/10.4995/CARMA2024.2024.17783
Espinosa, & Zúñiga. (2020). Aplicación de algoritmos Random Forest y XGBoost en la predicción de la inflación en México. Ingeniería, Investigación y Tecnología, 1-16. DOI: https://doi.org/10.22201/fi.25940732e.2020.21.3.022
Forte, A. (Septiembre de 2024). Inflación de corto plazo con random forest (Documento de trabajo No. 24/10). BBVA Research. Obtenido de file:///D:/Courses%20taken/Pron%C3%B3sticos%20Macro%20Fin/Modulo%205.%20Taller%20de%20investigacion/Papers/A.%20Random-forest%20Argentina.pdf
Hastie, T., Tibshirani, R., & Friedman, J. (2009). The Elements of Statistical Learning: Data Mining, Inference, and Prediction. Springer. DOI: https://doi.org/10.1007/978-0-387-84858-7
Hyndman, R. J., & Athanasopoulos, G. (2018). Forecasting: Principles and Practice. . OTexts.
Medeiros, M. C., Vasconcelos, G. F., Veiga, Á., & Zilberman, E. (2021). Forecasting inflation in a data-rich environment: The benefits of machine learning models. Journal of Business & Economic Statistics, 39(1), 98-119. DOI: https://doi.org/10.1080/07350015.2019.1637745
Medina Merino, R. F., & Ñique Chacón, C. I. (2017). Bosques aleatorios como extensión de los árboles de clasificación para la predicción de la inflación en Perú. Inter Fases, 10, 165-180. DOI: https://doi.org/10.26439/interfases2017.n10.1775
Meuller, S. (2022). Improving Inflation Forecast Accuracy with Random Forest Models. Universidad de Lund, Departamento de Economía.
Moyo, T., & Musengezi, J. (2022). Utilizing decision trees and random forests for inflation forecasting in African economies. . Journal of Economic Forecasting, 58(3), 345-359.
Patton, A. J., & Simsek, Y. (2023). Generalized autoregressive score trees and forests in economic predictions. International Journal of Forecasting, 41(4), 221-238. . DOI: https://doi.org/10.2139/ssrn.4459756
Pedregosa, F., Varoquaux, G., Gramfort, A., Michel, V., Thirion, B., Grisel, O., . . . Duchesnay, E. (2011). Scikit-learn: Machine learning in Python. Journal of Machine Learning Research, 12, 2825–2830.
Quinlan, J. R. (1986). Induction of Decision Trees. . Machine Learning., 1(1), 81-106. DOI: https://doi.org/10.1007/BF00116251
Silva, R. F., & Oliveira, M. A. (2019). Uso de Random Forest para la predicción de la inflación en Brasil. Revista Brasileira de Agroinformática, 15(2), 45-58.
Zhao, L., & Zhang, X. (2019). A Comparison of ARIMA and LSTM in Forecasting Time Series. IEEE Xplore.
Zhou, S., & Mentch, L. (2023). Trees, forests, and optimal pruning techniques in inflation forec
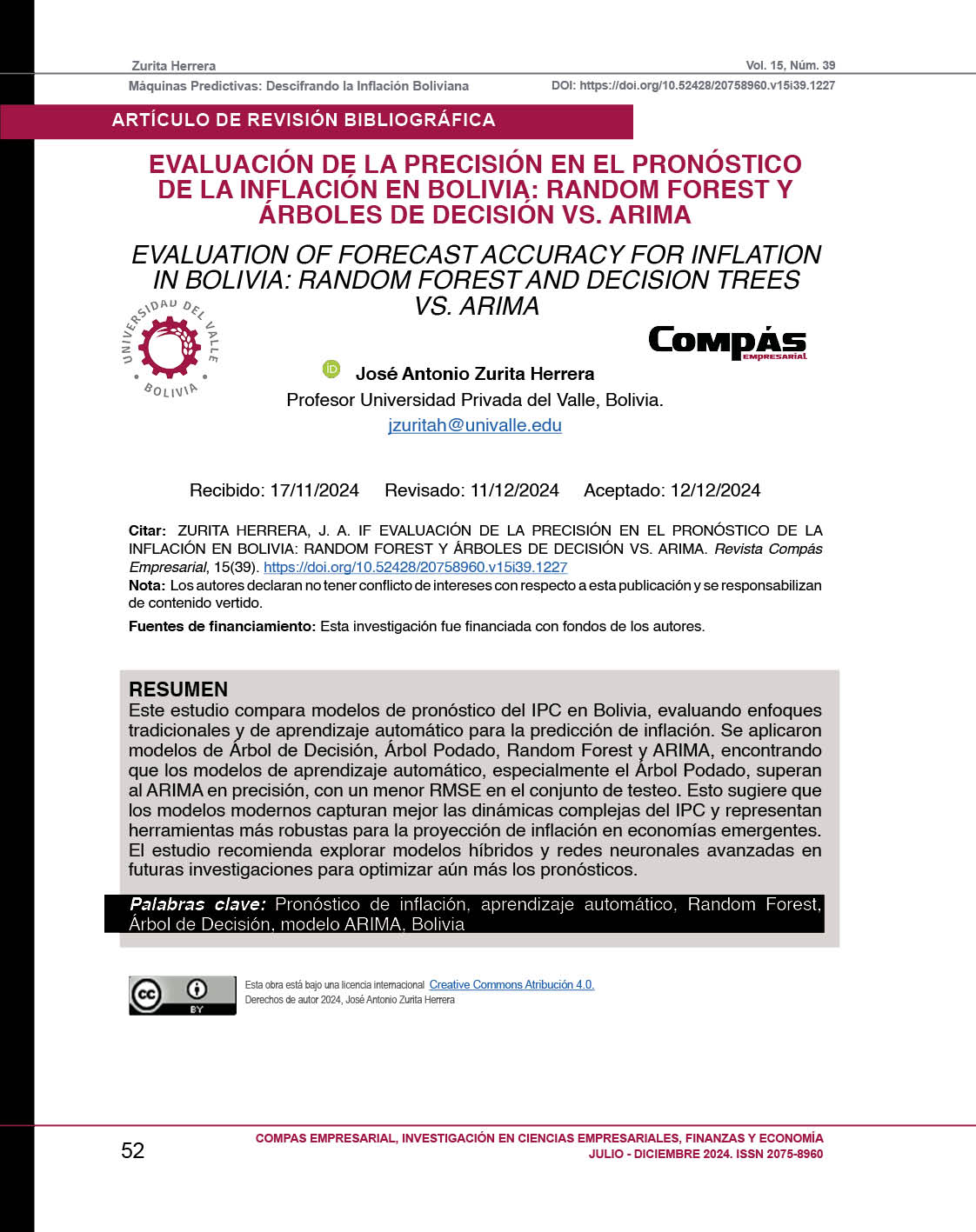
Published
How to Cite
Issue
Section
License
Copyright (c) 2024 JOSE ANTONIO ZURITA HERRERA

This work is licensed under a Creative Commons Attribution 4.0 International License.
Authors who publish with this journal agree to the following terms:
- Authors retain copyright and grant the journal right of first publication with the work simultaneously licensed under a Creative Commons Attribution License 4.0 that allows others to share the work with an acknowledgement of the work's authorship and initial publication in this journal.
- Authors are able to enter into separate, additional contractual arrangements for the non-exclusive distribution of the journal's published version of the work (e.g., post it to an institutional repository or publish it in a book), with an acknowledgement of its initial publication in this journal.
- Authors are permitted and encouraged to post their work online (e.g., in institutional repositories or on their website) prior to and during the submission process, as it can lead to productive exchanges, as well as earlier and greater citation of published work.