Modeling the Behavior of a Power Amplifier for 5G Using Machine Learning
DOI:
https://doi.org/10.52428/20758944.v20i55.1101Keywords:
Machine Learning, ADS, Amplificador, Modelado, 5GAbstract
The main purpose of this research is to model the behavior of a radio frequency (RF) amplifier using machine learning techniques. To achieve this goal, we use Keysight's ADS software. First, relevant data from graphs is obtained and transformed into tabular data. This data is essential for training the model. The obtained results show a remarkable agreement with the expected behavior of the RF amplifier, which confirms the effectiveness of the proposed approach. This finding not only validates the feasibility of modeling amplifiers, but also suggests the applicability of these techniques in the modeling of diverse electronic systems with a high degree of accuracy and reliability.
Downloads
References
Colzani, A., Fumagalli, M., Fonte, A., Traversa, A., & Ture, E. (2022, September). Long-Reach
E-band HPA for 5G Radio Link. In 2022 52nd European Microwave Conference (EuMC) (pp. 760-763). IEEE.
Dikmese, S., Anttila, L., Campo, P. P., Valkama, M., & Renfors, M. (2019, August). Behavioral modeling of power amplifiers with modern machine learning techniques. In 2019 IEEE MTT-S https://doi.org/10.1109/IMC-5G47857.2019.9160381
International Microwave Conference on Hardware and Systems for 5G and Beyond (IMC-5G)(pp. 1-3). IEEE.
Huo, Y., Dong, X., & Xu, W. (2017). 5G cellular user equipment: From theory to practical
https://doi.org/10.1109/ACCESS.2017.2727550
https://doi.org/10.1109/ACCESS.2017.2727550 hardware design. IEEE Access, 5, 13992-14010.
https://doi.org/10.1109/ACCESS.2017.2727550
Ji, H. G., Jeong, J. H., & Kang, D. M. (2023). Balanced GaN HPA MMIC for 5G FR2 Band Base
https://doi.org/10.5515/KJKIEES.2023.34.6.444
https://doi.org/10.5515/KJKIEES.2023.34.6.444
https://doi.org/10.5515/KJKIEES.2023.34.6.444
Station. The Journal of Korean Institute of Electromagnetic Engineering and Science, 34(6), 444-449.
Liu, B., Deferm, N., Zhao, D., Reynaert, P., & Gielen, G. G. (2012). An efficient high-frequency linear RF amplifier synthesis method based on evolutionary computation and machine learning techniques. IEEE Transactions on Computer-Aided Design of Integrated Circuits and Systems, 31(7), 981-993. https://doi.org/10.1109/TCAD.2012.2187207
Ma, R., Benosman, M., Manjunatha, K. A., Komatsuzaki, Y., Shinjo, S., Teo, K. H., & Orlik, P.
V. (2018, August). Machine-learning based digital Doherty power amplifier. In 2018 IEEE International Symposium on Radio-Frequency Integration Technology (RFIT) (pp. 1-3). IEEE.
Mounir, M., El Mashade, M. B., Aboshosha, A. M., & Youssef, M. I. (2022). Impact of HPA nonlinearity on the performance of power domain OFDM-NOMA system. Engineering Research Express, 4(2), 025004 https://doi.org/10.1088/2631-8695/ac5aa2
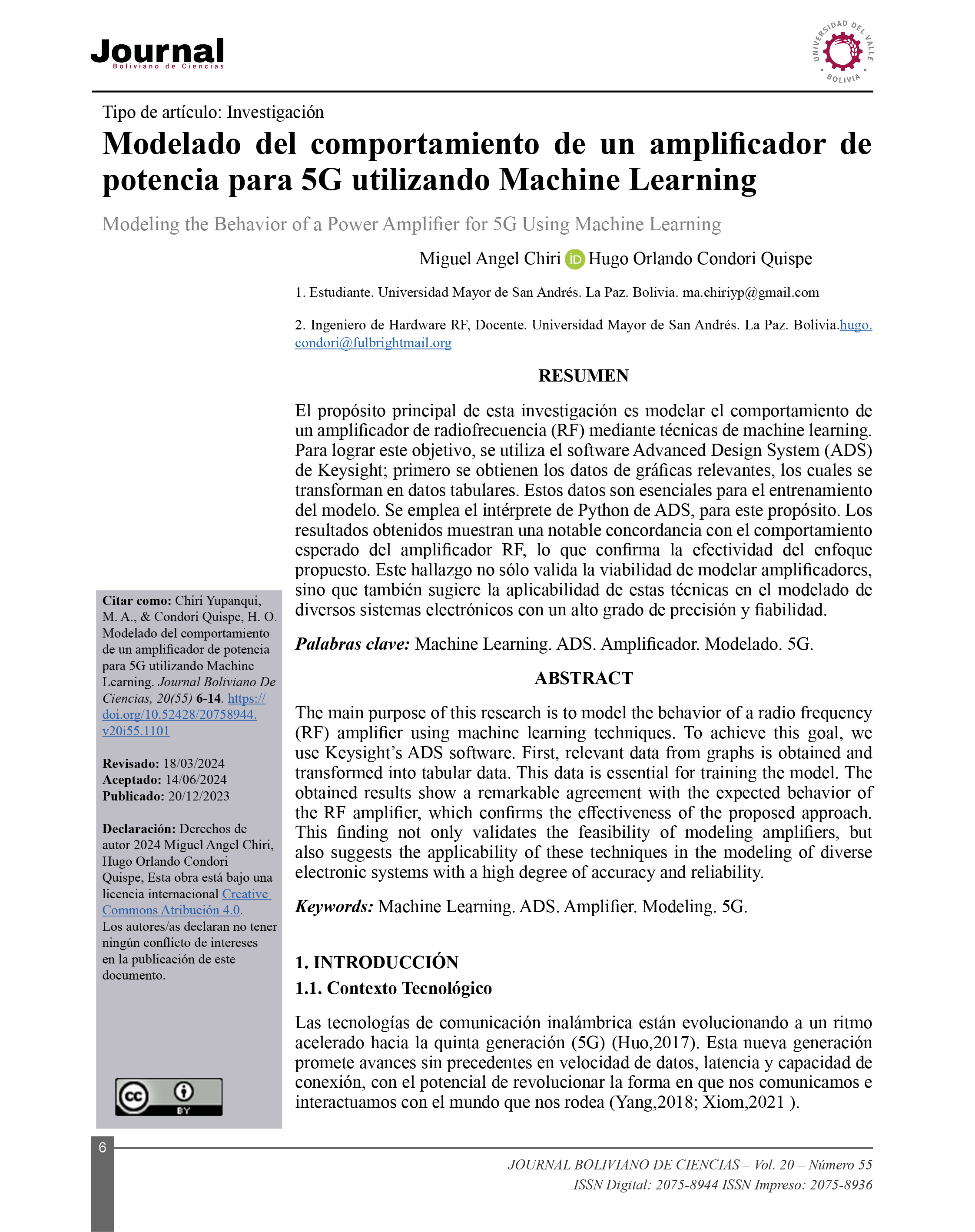
Published
How to Cite
Issue
Section
License
Copyright (c) 2024 Miguel Angel Chiri Yupanqui, Hugo Orlando Condori Quispe

This work is licensed under a Creative Commons Attribution 4.0 International License.
Authors who publish with this journal agree to the following terms:
- Authors retain copyright and grant the journal right of first publication with the work simultaneously licensed under a Creative Commons Attribution License 4.0 that allows others to share the work with an acknowledgement of the work's authorship and initial publication in this journal.
- Authors are able to enter into separate, additional contractual arrangements for the non-exclusive distribution of the journal's published version of the work (e.g., post it to an institutional repository or publish it in a book), with an acknowledgement of its initial publication in this journal.
- Authors are permitted and encouraged to post their work online (e.g., in institutional repositories or on their website) prior to and during the submission process, as it can lead to productive exchanges, as well as earlier and greater citation of published work.